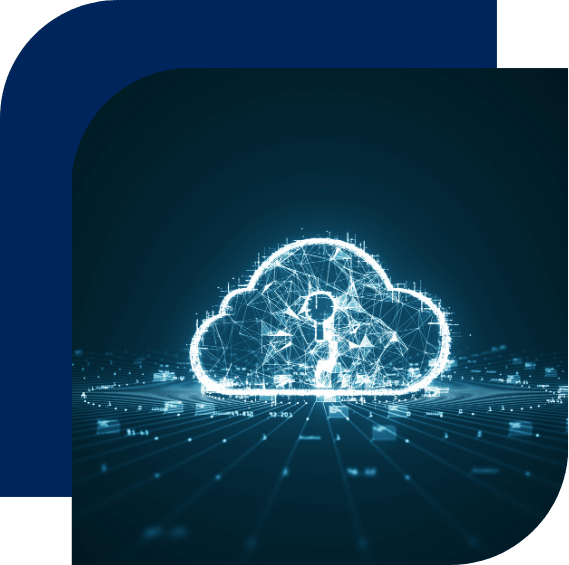
Data, Visualisation
& AI
Data is the engine of an organisation. Learn to use your data properly and maximise insights whilst driving operational growth.
Mobilise will make your data work for you.
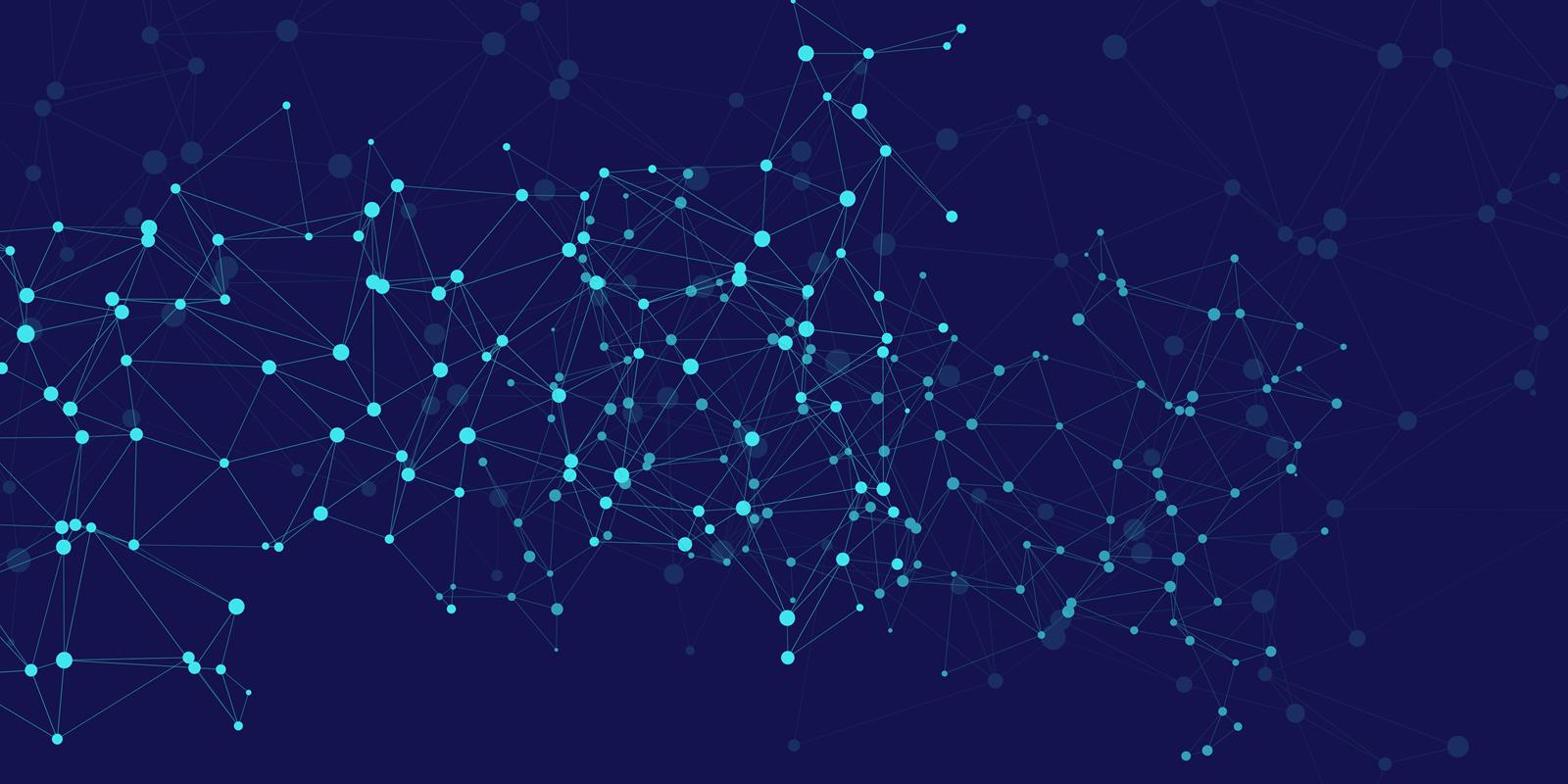
Understand, learn, and predict with business intelligence insights.
Mobilise understand that managing data can be overwhelming and confusing, which is why we’re here to help.
Expert Assistance
For Organisational Success
Data transformation is a powerful organisational activity. It allows you to turn pools of shapeless data into powerful insights and develops your enterprise with advanced AI technology.
Mobilise Empowers the Crown Prosecution Service with Modern Data Analytics Platform.
Are you are struggling to extract value from siloed data sources and outdated legacy platforms?
The Crown Prosecution Service (CPS) faced the same challenge, but they found a solution with Mobilise. We deployed a talented team of experts, including User Researchers, SCRUM Masters, Data Architects, Data Analysts, and DevOps Engineers to build a modern data analytics platform on Azure.
Our strategy included applying data validation, securing access, and ensuring new data products met strict requirements before being onboarded, allowing CPS to make data driven decisions.
We then helped CPS establish a data catalogue that improved the understanding of their available data, empowering them with great expertise in their datasets.
Using a modular approach, we continually refined ingestion, data, middleware, and ingestion components, which resulted in a 3000% improvement in PowerBI load times, among other benefits. This was supported by a managed service and the enablement of CPS staff with custom training materials, knowledge transfer workshops, paired programming, and remotely recorded sessions.
Now, CPS has a modern data analytics platform that aggregates data from multiple business areas, across different technologies, providing a secure, flexible data environment to drive business analysis and decision-making through automation and best practice. Mobilise has helped CPS become a data-driven organization by transforming technology, data, people, and practices. Don’t let your data go to waste – let Mobilise help you unlock its potential.
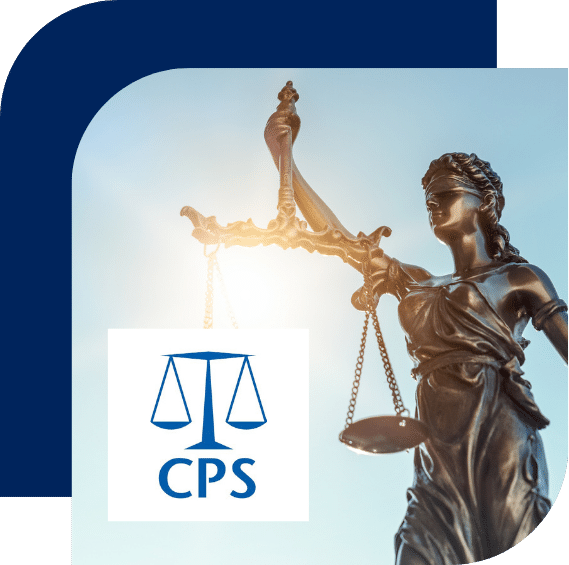
Data Services
Our data experts can guide you through the data transformation journey. Mobilise enable organisations, growing the potential of your data through smart insights, data management, and the building of a Data Centre of Excellence.
Mobilise want you to know your data and believe that data should be accessible to everyone. Our experts will support your people via our developed Knowledge Transfer Framework, allowing you to see the benefits of transformation as it happens and into the future
Data Expertise
Our data experts have a deep knowledge base enabling them to support and deliver:
- Data Health Check & Gap Analysis
- Data Maturity Assessments
- Data Management & Restructuring
- Data Enablement & Resource
- Upskilling
- Data Strategy & Development
- Data Platform Development & Build
- Data Centre of Excellence Transformations
Mobilise are – Microsoft Solutions Partners for Data & AI (Azure), Elastic Search Partners, and a Power BI trained organisation.

Abri, one of the UK's largest housing association had the goal of becoming a data driven organisation.
The results of our delivery can be seen by looking at our journey with Abri, a housing association in the South of England. As one of the UK’s largest housing associations, with 35,000 homes and over 100,000 residents, data security was crucial, and they had a strategic goal of becoming a data driven organisation.
Mobilise helped make this goal a reality by supporting large-scale data transformation. We are proud to have made Abri’s data infrastructure more secure and dynamic, building out their existing Azure-based data landscape and enabling Abri to maintain their own infrastructure.
Along this journey we embedded our experts into Abri’s in-house team, outlining the steps needed to upskill existing resources to ensure Abri’s independence and the long-term sustainability of the data infrastructure.
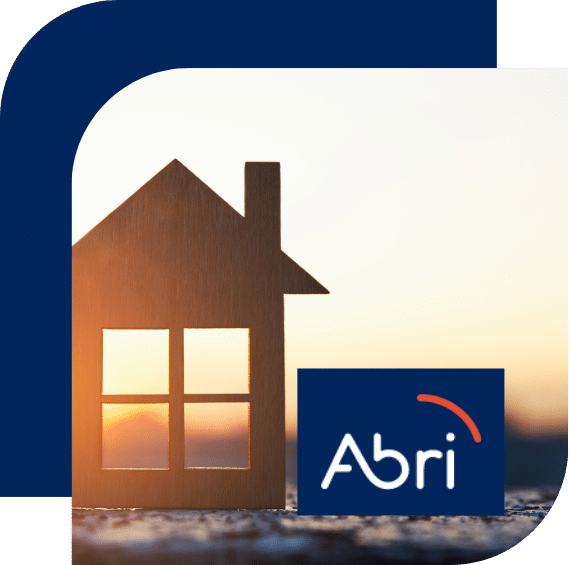
Mobilise cuts through the jargon to bring the benefits of AI, automation and cutting-edge interfaces to your organisation
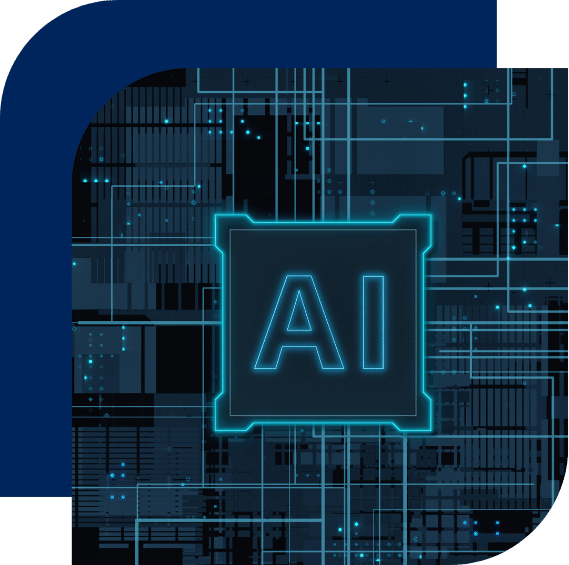
Artificial Intelligence Services
Artificial Intelligence (AI) has come a long way in recent years. Mobilise leverages powerful AI that can help build new business capabilities and manage the relationships between data, platforms, software, and applications.
The DVLA now has a wealth of information available to them from millions of events, visualised using custom-built Power BI Dashboards.
Due to the success of the initial implementation, the DVLA is looking to expand the MIBI solution to other areas of the agency.
In 2020, the Driver and Vehicle Licensing Agency (DVLA) faced a challenge. They lacked the data necessary to analyse trends, report to the motor industry, and make accurate vehicle-related forecasts. To overcome this, they embarked on a project with Mobilise to aggregate event data into a central data store, enabling secure data analysis across business units.
Mobilise was embedded into the DVLA’s Management Information and Business Intelligence (MIBI) delivery team to design, build, and support a centralised solution.
We used Agile methodologies to estimate and prioritise work, collaborated with DVLA Business Analysts and Data Architects to build a backlog of work items, and identified milestones to roadmap the delivery of MIBI.
By partnering with Mobilise, the DVLA has transformed their data architecture, unlocking valuable insights to drive their business forward.
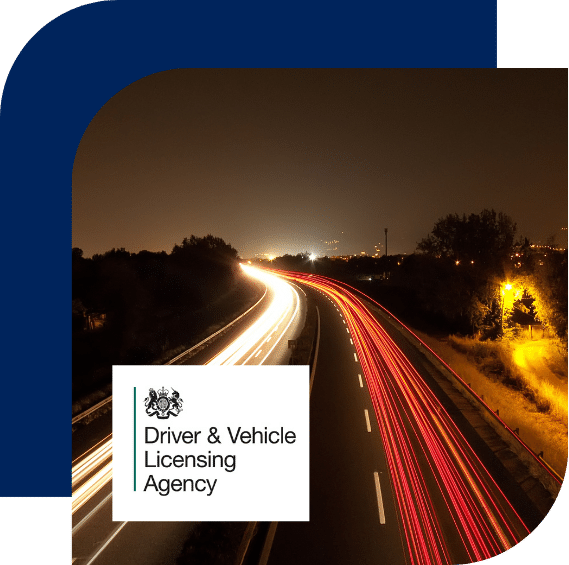
Data Centre of Excellence Services
A DCoE means investing in and nurturing organic growth while maintaining governance and control
A Data Centre of Excellence (DCoE) at the heart of all things data. As organisations manage exponentially growing data landscapes, there’s an increased demand for insights, data-management, and sustainable governance. A Data CoE can be the key to success.
Managing your data landscape and mobilising the correct insights could be the difference to meeting, surpassing, or missing your set strategic goals. If you can effectively manage and exploit your data, organisational goals become more attainable and processes more visible.
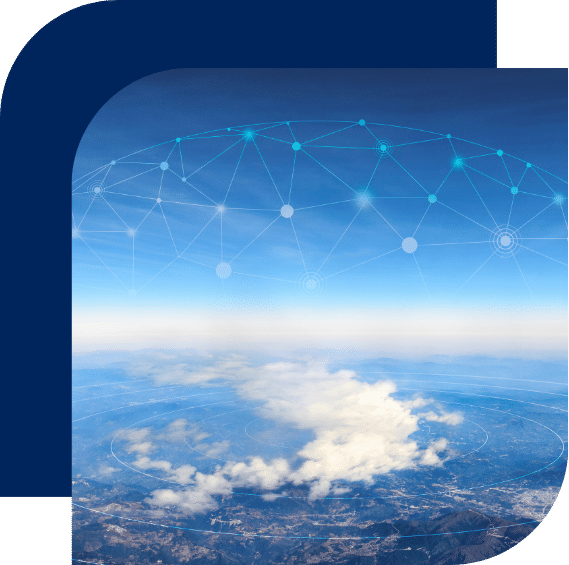
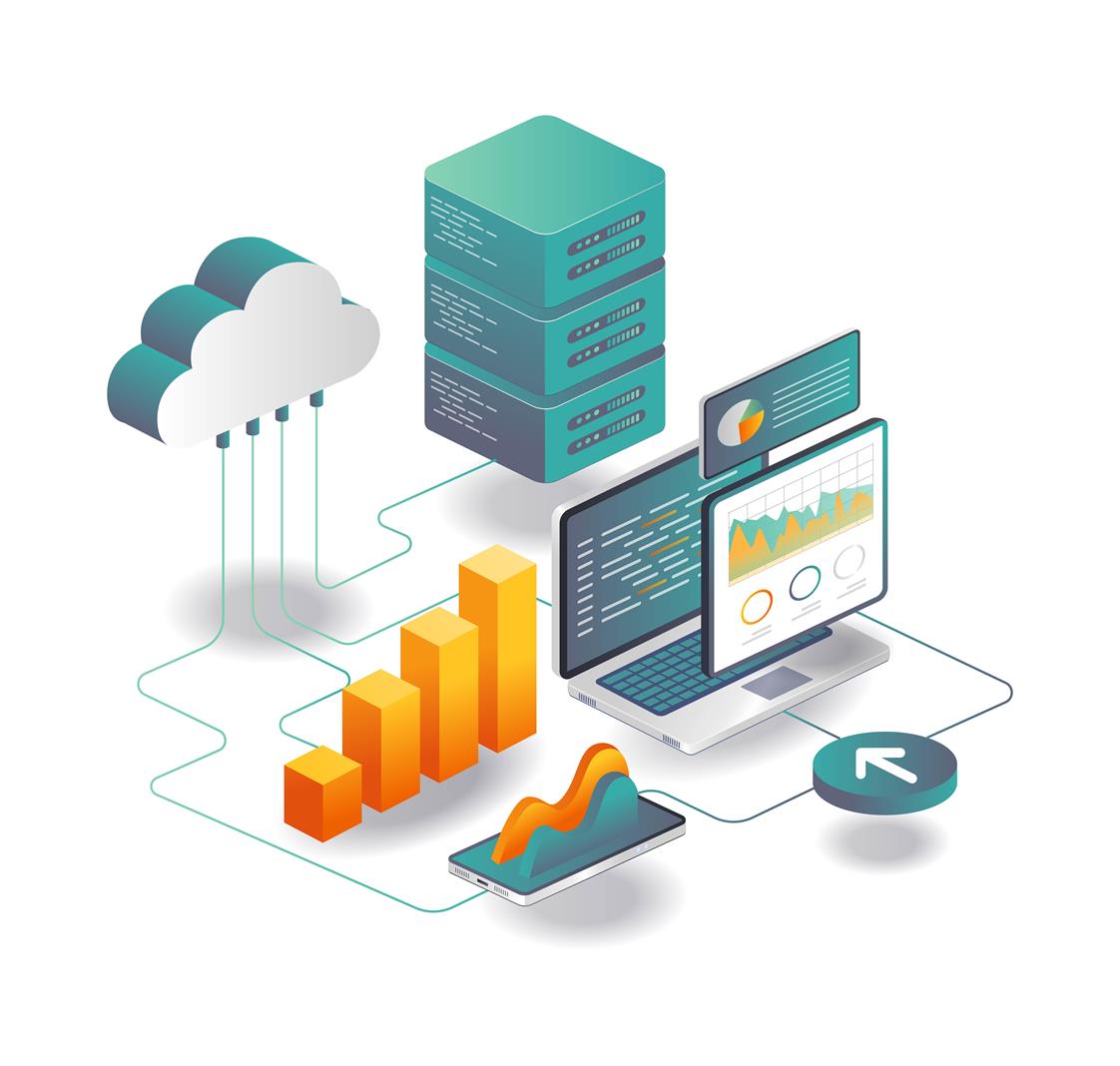
As a central function a CoE is a powerful way to break down geographic and organisational silos.
This allows you to align around operational goals rather than individual department metrics.
Mobilise will build your CoE to fit your existing technology landscape. If you are a majority Microsoft organisation, we will build a Microsoft Power Platform Centre of Excellence.
We will help you establish continuous Insights for your delivery of services.
These will provide live updates on organisational performance indicators such as:
- Demand
- Sales performance
- Customer/client behaviour
- Stakeholder activity
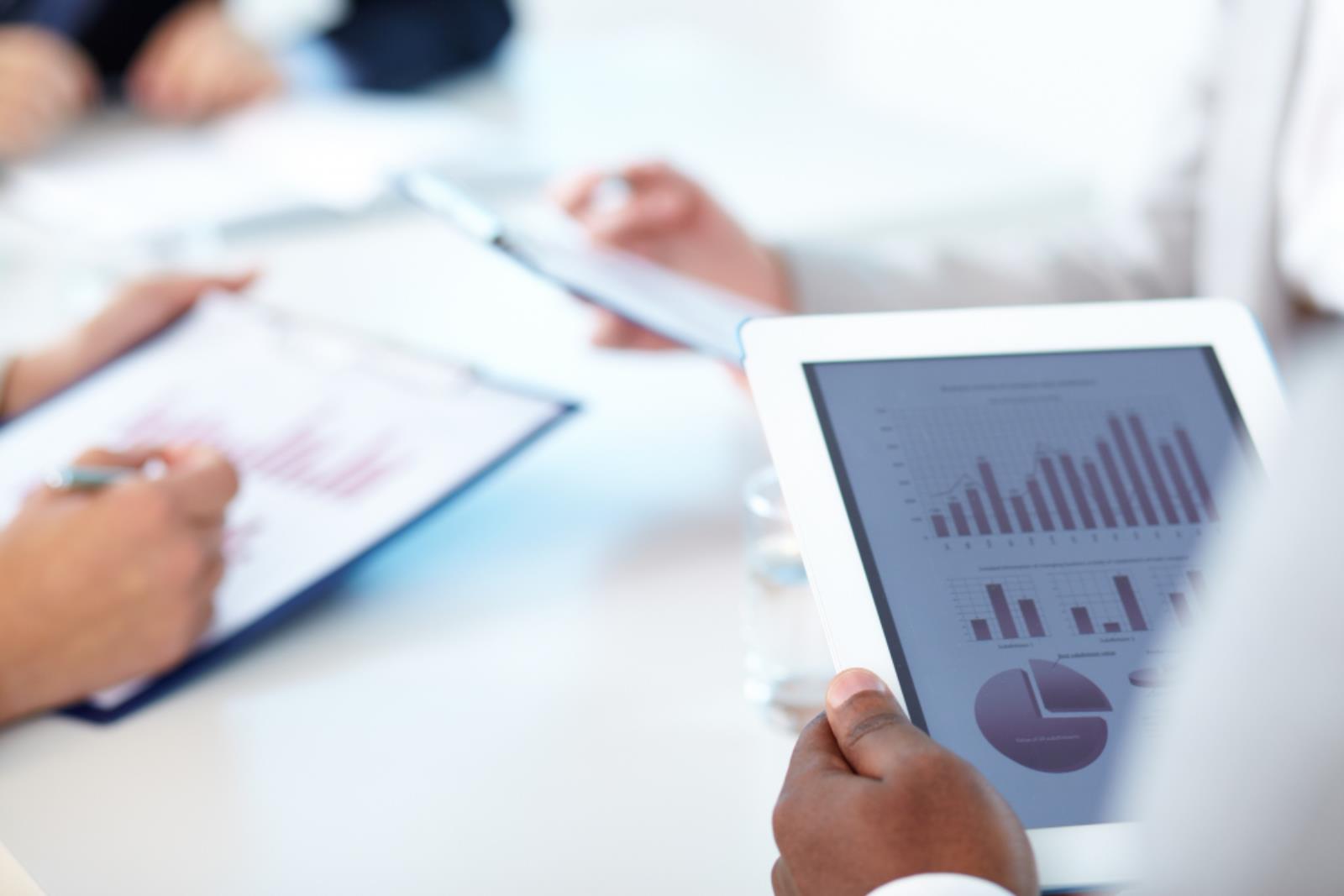
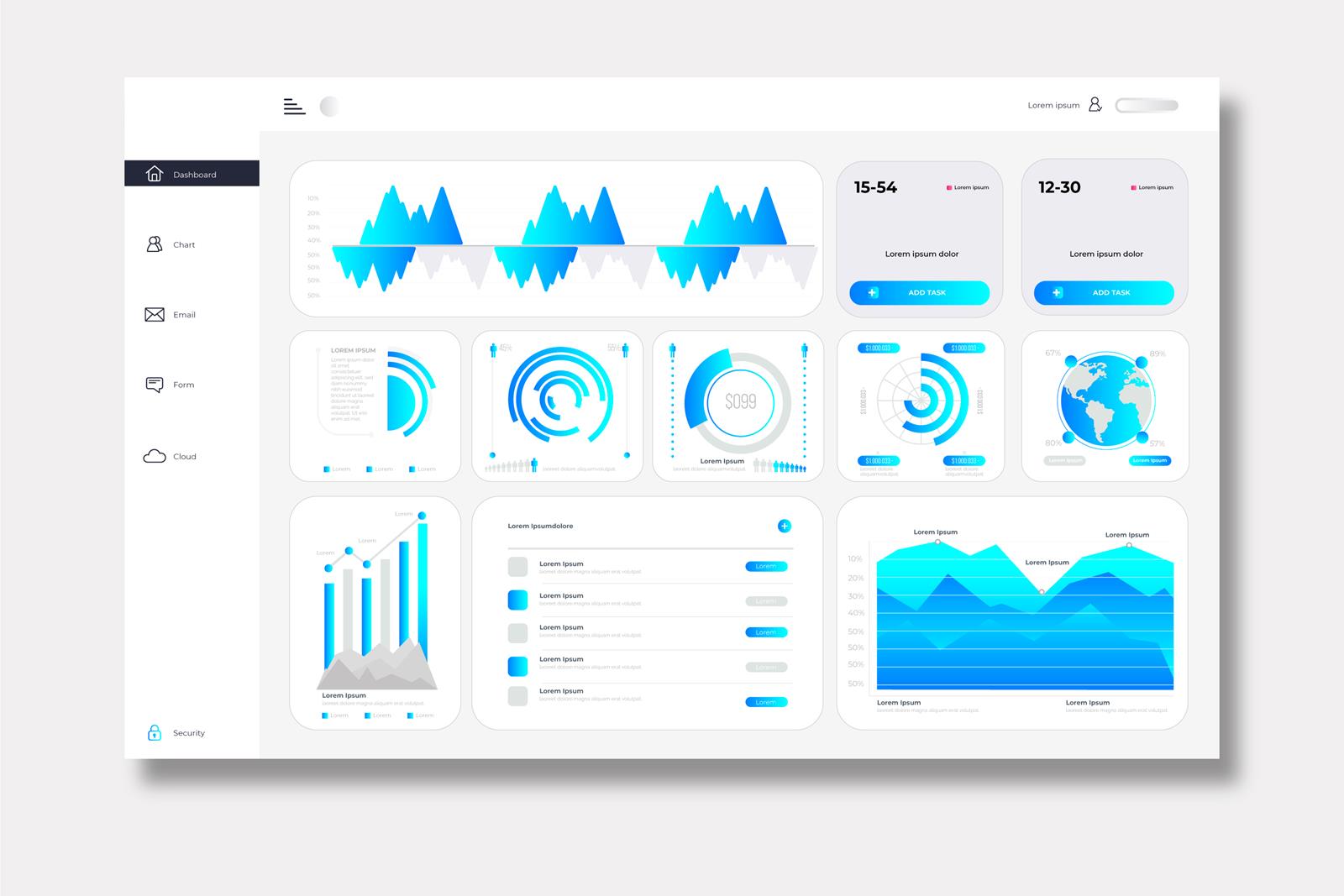
The Key Data CoE Steps:
Data influences every part of an organisation. Recognising your common data pain points will allow you to focus on these core issues and break down silos between functions.
An agile data plan, designed by solutions architects, will make sure projects can be delivered at pace. This will allow time for enablement so that your people can learn the new landscape and reap the rewards.
Upskilling and ensuring the right tools are in place is crucial. This means building a healthy data culture throughout the organisation, earning buy-in from key stakeholders and promoting the support of leadership.
Change is constant in technology; you must build in continuous learning functions and processes to ensure adaptation. This way your data landscape will continue to grow and will resist becoming stationary or outdated.