Data Warehouse vs Data Lake
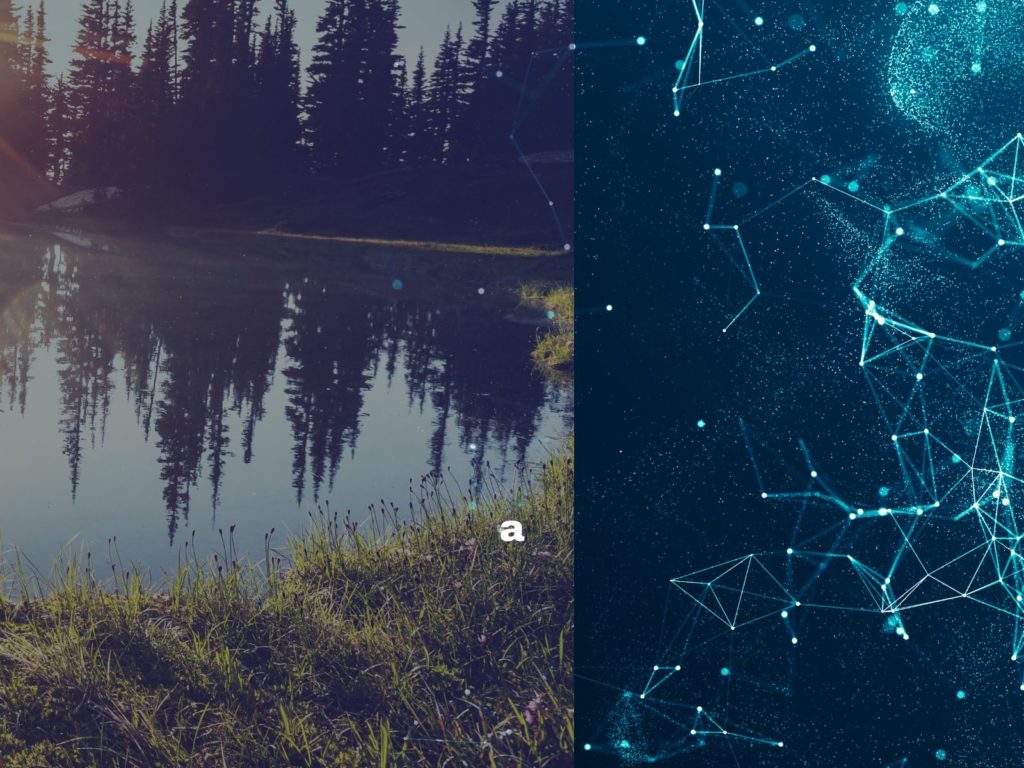
Differences
Data
Data warehouses are different in terms of how they can save the data added to them. They can only incorporate data that has been designed in a structured manner, whereas conversely, a Data Lake can store raw data. This means that it can include data with no structure, is semi-structured, or is entirely natural. It is, therefore, more useful for engineers because they can store what is referred to as hot and cold data.
Processing
The processing abilities of both database storage management systems are different. You must index data into an acceptable design structure to incorporate it into a data warehouse. It means that you will be able to access it only through a schema. A schema is a predetermined form of data acceptance that the warehouse will have been programmed with.
Data Lakes offer a completely different approach because they can take data as it comes. This means that it is stored as-is. However, when you now want to perform analytics on the data, you must provide a schema. Therefore, the difference between these two terms of processing lines within when you must structure the data. A Data Lake does not require direct structuring; however, a database warehouse does.
Cost
Cost is an important consideration depending on the needs and wants of your departments. Large organisations that require big data technologies have found that the custom storage abilities of different systems make a huge difference to the efficiency of the Departments in terms of budgets.
Data warehouses are often more expensive than Data Lakes, and this is because of the licensing and support that is involved when developing a schema. Since a Data Lake can make use of low-cost commodity hardware, you will find that it often brings down the price of the overall usage of the software. This is because the data has not been structured and is merely being stored. The analytics component of a database warehouse is what makes it more expensive than its competitor.
Flexibility
The warehouse has been designed in such a way that it cannot operate loosely. It is a structured data bank that means that you must work within the predetermined configuration to use it. Therefore, it has the least flexibility between the two options we are discussing here today.
If you decide that you would like to change the structure, this is still possible because the manufacturers have not made it that rigid. What you will require, however, is quite a large amount of technical capability within your organisation because getting this wrong could lead to large amounts of data being stored incorrectly. It will also take quite a lot of time away from the technical professionals handling this.
On the other hand, you will find that a Data Lake has pretty much got no structure. The positive of this is that it allows your data scientists to design the data schema for themselves, meaning that they can customise it to their own needs. The negative side of this is that if you are looking for something readymade, this is not a good option.
Use Cases
The following use cases highlight the differences between the two. As its name suggests, data warehouses are designed to store and retrieve large volumes of data. The warehouse environment is often built to handle multiple unstructured data types. Data warehouses are usually made in such a way that they can analyse massive amounts of data. The warehouse is often integrated with other enterprise applications and data sources for the necessary administration and maintenance.
Data Lakes are designed to accept all sorts of data at the rate it is produced. Data Lakes are designed to handle unstructured data and to store it efficiently. Data Lakes are usually used to store big data, generally smaller than a terabyte, so that you can compare it with other data sources later.
When To Use Data Warehouse instead of Data Lakes
A data warehouse is practical when a company has multiple legacy systems connected with its real-time system. It is also useful when the company requires access to real-time data and requires a centralised view of its needs. Data Lakes are helpful when a company requires data to be used for specific purposes, such as using historical data to allow the future analysis of trends.
When To Use Data Lakes instead of Data Warehouse
A Data Lake is valuable when companies want to store big data and analyse it unsupervised. It is also useful when the company has many different data sources.
Data Lakes represent a significant development in data management and can be used in multiple ways. However, they are not suitable for all types of data or business use cases. The organisation that builds such a data lake must understand its needs and the various issues and requirements it will encounter soon while building an enterprise data lake.
Data Lake Software
Data Lake management software programs automate many of the processes, and in this way, they can speed up the data lake development process. These are some of the commonly used data lake software.
Data Lake Tools are more focused on the design and development of a Data Lake, while Data Lake Management Tools focus more on the management and monitoring of a data lake. This is how this difference is defined.
Data Warehouse Software
Simply put, a data warehouse is a centralised repository of data used by multiple applications and users. It helps the organisation by allowing them better to understand their product or service and its customers. Data warehouses often store large datasets.
They are used to build reports and analyse trends that would otherwise be difficult to find if the data was spread through various databases and applications. A data warehouse is a collection of data stored in a single database copied or transformed from one or more operational systems.
Conclusion
In conclusion, you will find that these two database systems are entirely different in saving information. When dealing with the warehouse, you will find that information needs to be structured in a particular manner for it to be accepted.
An example of this would be that you cannot just put in a combination of text and numbers if the structure were designed for only numbers. If, for example, you are building a database that requires the input of birthdays in the format of a certain number, then you will not be able to add words.
When looking at data lakes, you will have complete power over what data you like to input and how. You will be able to input text or numbers. The only difference here is that you shall struggle in terms of the analytics you will perform because the data at some point will need to become structured for you to analyse it.